在神经网络中,不同任务类型(如多分类、二分类、回归)需要使用不同的损失函数来衡量模型预测和真实值之间的差异。选择合适的损失函数对于模型的性能至关重要。
这里的是API 的注意⚠️,但是在真实的公式中,目标值一定是热编码之后的,但是在API中可以是热编码之前的。
热编码指的是:假设一个目标值是【0,1,2,3,4】
热编码是,默认会找你的最大值去,确定有多少个0,因为0也算一个位置,所以如果最大值为5,那么就一共有6位(0,1,2,3,4,5
)
# 多分类的损失,热编码之前import torch
import torch.nn as nn
# 真实值
y_true = torch.tensor([2,3],dtype=torch.int64)
y_predict = torch.tensor([[10,20,35,20,23],[23,22,22,26,12]],dtype=torch.float32)# 损失计算
loss = nn.CrossEntropyLoss()
print(loss(y_predict,y_true))
tensor(0.0414)
#多分类损失,热编码之后
import torch
import torch.nn as nn
# 真实值
# y_true = torch.tensor([2,3],dtype=torch.int64)
y_true= torch.tensor([[0,0,1,0],[0,0,0,1]],dtype=torch.float32)
y_predict = torch.tensor([[10,20,35,20],[23,22,22,26]],dtype=torch.float32)# 损失计算
loss = nn.CrossEntropyLoss()
print(loss(y_predict,y_true))
tensor(0.0414)
# 二分类的损失import torch
import torch.nn as nn
# 真实值
y_true = torch.tensor([0,0,1],dtype=torch.float32)# 预测值
y_predict= torch.tensor([0.2,0.1,0.8],dtype=torch.float32)# 损失计算
loss = nn.BCELoss()
print(loss(y_predict,y_true))
tensor(0.1839)
L1 这个损失函数最大的特点是: 零点不平滑,导致不可导,跳过极小值,所以不会用来做损失函数,而是做正则化用来缓解过拟合。
L2 的特点是,当初始值的给的不好,导致预测值和目标值差异大的时候,会产生梯度爆炸,所以我们也不用这个损失函数,而是做正则化来缓解过拟合。
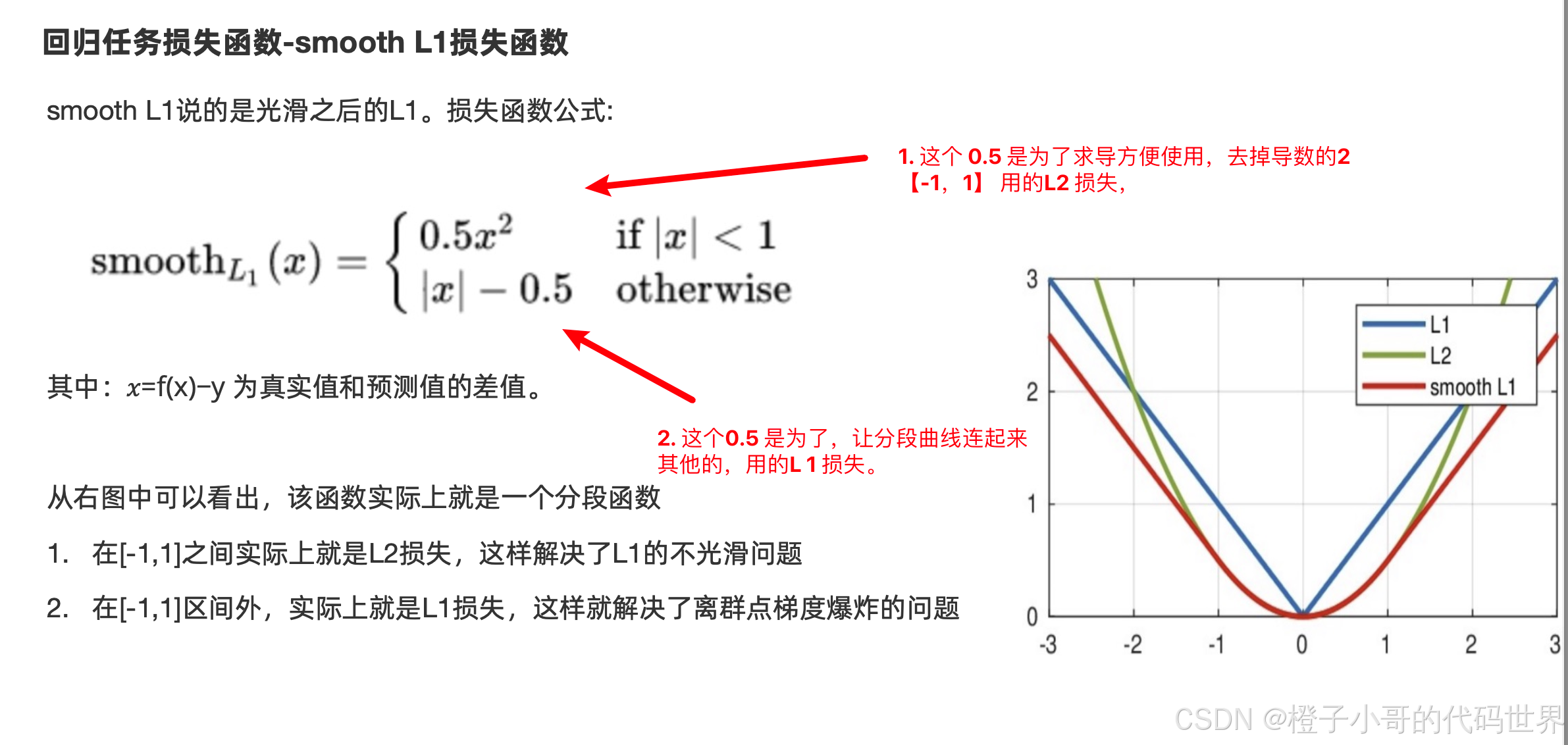
把L1 和 L2 损失函数,联合起来。就是我们的 smooth L1 损失函数
import torch
import torch.nn as nn# 真实值
y_true = torch.tensor([1.0,2.0,3.0])# 预测值
y_predict= torch.tensor([2.0,2.5,5.0])# 损失计算
l1 = nn.L1Loss()
l2 = nn.MSELoss()
sml1 = nn.SmoothL1Loss()
print(l1(y_predict,y_true))
print(l2(y_predict,y_true))
print(sml1(y_predict,y_true))
对于回归任务建议使用的 SmoothL1 损失。