Python 机器学习 基础 之 处理文本数据 【停用词/用tf-idf缩放数据/模型系数/多个单词的词袋/高级分词/主题建模/文档聚类】的简单说明
目录
Python 机器学习 基础 之 处理文本数据 【停用词/用tf-idf缩放数据/模型系数/多个单词的词袋/高级分词/主题建模/文档聚类】的简单说明
一、简单介绍
二、停用词
三、用tf-idf缩放数据
四、研究模型系数
五、多个单词的词袋(n 元分词)
六、高级分词、词干提取与词形还原
七、主题建模与文档聚类
1、隐含狄利克雷分布
附录:
一、参考文献
二、spacy 环境配置
一、简单介绍
Python是一种跨平台的计算机程序设计语言。是一种面向对象的动态类型语言,最初被设计用于编写自动化脚本(shell),随着版本的不断更新和语言新功能的添加,越多被用于独立的、大型项目的开发。Python是一种解释型脚本语言,可以应用于以下领域: Web 和 Internet开发、科学计算和统计、人工智能、教育、桌面界面开发、软件开发、后端开发、网络爬虫。
Python 机器学习是利用 Python 编程语言中的各种工具和库来实现机器学习算法和技术的过程。Python 是一种功能强大且易于学习和使用的编程语言,因此成为了机器学习领域的首选语言之一。Python 提供了丰富的机器学习库,如Scikit-learn、TensorFlow、Keras、PyTorch等,这些库包含了许多常用的机器学习算法和深度学习框架,使得开发者能够快速实现、测试和部署各种机器学习模型。
Python 机器学习涵盖了许多任务和技术,包括但不限于:
- 监督学习:包括分类、回归等任务。
- 无监督学习:如聚类、降维等。
- 半监督学习:结合了有监督和无监督学习的技术。
- 强化学习:通过与环境的交互学习来优化决策策略。
- 深度学习:利用深度神经网络进行学习和预测。
通过 Python 进行机器学习,开发者可以利用其丰富的工具和库来处理数据、构建模型、评估模型性能,并将模型部署到实际应用中。Python 的易用性和庞大的社区支持使得机器学习在各个领域都得到了广泛的应用和发展。
二、停用词
删除没有信息量的单词还有另一种方法,就是舍弃那些出现次数太多以至于没有信息量的单词。有两种主要方法:使用特定语言的停用词(stopword)列表,或者舍弃那些出现过于频繁的单词。scikit-learn
的 feature_extraction.text
模块中提供了英语停用词的内置列表:
from sklearn.feature_extraction.text import ENGLISH_STOP_WORDS
print("Number of stop words: {}".format(len(ENGLISH_STOP_WORDS)))
print("Every 10th stopword:\n{}".format(list(ENGLISH_STOP_WORDS)[::10]))
Number of stop words: 318 Every 10th stopword: ['up', 'upon', 'can', 'even', 'few', 'could', 'whenever', 'though', 'system', 'seem', 'how', 'always', 'whereas', 'perhaps', 'rather', 'ltd', 'will', 'became', 'thru', 'if', 're', 'last', 'yet', 'anyone', 'become', 'although', 'from', 'four', 'find', 'it', 'etc', 'two']
显然,删除上述列表中的停用词只能使特征数量减少 318 个(即上述列表的长度),但可能会提高性能。我们来试一下:
# 指定stop_words="english"将使用内置列表。
# 我们也可以扩展这个列表并传入我们自己的列表。
vect = CountVectorizer(min_df=5, stop_words="english").fit(text_train)
X_train = vect.transform(text_train)
print("X_train with stop words:\n{}".format(repr(X_train)))
X_train with stop words: <25000x26966 sparse matrix of type '<class 'numpy.int64'>'with 2149958 stored elements in Compressed Sparse Row format>
现在数据集中的特征数量减少了 305 个(27271-26966),说明大部分停用词(但不是所有)都出现了。我们再次运行网格搜索:
grid = GridSearchCV(LogisticRegression(), param_grid, cv=5)
grid.fit(X_train, y_train)
print("Best cross-validation score: {:.2f}".format(grid.best_score_))
Best cross-validation score: 0.88
使用停用词后的网格搜索性能略有下降——不至于担心,但鉴于从 27 000 多个特征中删除 305 个不太可能对性能或可解释性造成很大影响,所以使用这个列表似乎是不值得的。固定的列表主要对小型数据集很有帮助,这些数据集可能没有包含足够的信息,模型从数据本身无法判断出哪些单词是停用词。作为练习,你可以尝试另一种方法,即通过设置 CountVectorizer
的 max_df
选项来舍弃出现最频繁的单词,并查看它对特征数量和性能有什么影响。
三、用tf-idf缩放数据
另一种方法是按照我们预计的特征信息量大小来缩放特征,而不是舍弃那些认为不重要的特征。最常见的一种做法就是使用词频 - 逆向文档频率 (term frequency - inverse document frequency,tf-idf)方法。这一方法对在某个特定文档中经常出现的术语给予很高的权重,但对在语料库的许多文档中都经常出现的术语给予的权重却不高。如果一个单词在某个特定文档中经常出现,但在许多文档中却不常出现,那么这个单词很可能是对文档内容的很好描述。scikit-learn
在两个类中实现了 tf-idf 方法:TfidfTransformer
和 TfidfVectorizer
,前者接受 CountVectorizer
生成的稀疏矩阵并将其变换,后者接受文本数据并完成词袋特征提取与 tf-idf 变换。tf-idf 缩放方案有几种变体,你可以在维基百科上阅读相关内容(https://en.wikipedia.org/wiki/Tf-idf )。单词 w 在文档 d 中的 tf-idf 分数在 TfidfTransformer
类和 TfidfVectorizer
类中都有实现,其计算公式如下所示(这里给出这个公式主要是为了完整性,你在使用 tf-idf 时无需记住它):
其中 N 是训练集中的文档数量,Nw 是训练集中出现单词 w 的文档数量,tf (词频)是单词 w 在查询文档 d (你想要变换或编码的文档)中出现的次数。两个类在计算 tf-idf 表示之后都还应用了 L2 范数。换句话说,它们将每个文档的表示缩放到欧几里得范数为 1。利用这种缩放方法,文档长度(单词数量)不会改变向量化表示。
由于 tf-idf 实际上利用了训练数据的统计学属性,所以我们将使用在之前介绍过的管道,以确保网格搜索的结果有效。这样会得到下列代码:
from sklearn.feature_extraction.text import TfidfVectorizer
from sklearn.pipeline import make_pipeline
pipe = make_pipeline(TfidfVectorizer(min_df=5),LogisticRegression())
param_grid = {'logisticregression__C': [0.001, 0.01, 0.1, 1, 10]}grid = GridSearchCV(pipe, param_grid, cv=5)
grid.fit(text_train, y_train)
print("Best cross-validation score: {:.2f}".format(grid.best_score_))
Best cross-validation score: 0.89
如你所见,使用 tf-idf 代替仅统计词数对性能有所提高。我们还可以查看 tf-idf 找到的最重要的单词。请记住,tf-idf 缩放的目的是找到能够区分文档的单词,但它完全是一种无监督技术。因此,这里的“重要”不一定与我们感兴趣的“正面评论”和“负面评论”标签相关。首先,我们从管道中提取 TfidfVectorizer
:
vectorizer = grid.best_estimator_.named_steps["tfidfvectorizer"]
# 变换训练数据集
X_train = vectorizer.transform(text_train)
# 找到数据集中每个特征的最大值
max_value = X_train.max(axis=0).toarray().ravel()
sorted_by_tfidf = max_value.argsort()
# 获取特征名称
feature_names = np.array(vectorizer.get_feature_names_out())print("Features with lowest tfidf:\n{}".format(feature_names[sorted_by_tfidf[:20]]))print("Features with highest tfidf: \n{}".format(feature_names[sorted_by_tfidf[-20:]]))
Features with lowest tfidf: ['suplexes' 'gauche' 'hypocrites' 'oncoming' 'songwriting' 'galadriel''emerald' 'mclaughlin' 'sylvain' 'oversee' 'cataclysmic' 'pressuring''uphold' 'thieving' 'inconsiderate' 'ware' 'denim' 'reverting' 'booed''spacious'] Features with highest tfidf: ['gadget' 'sucks' 'zatoichi' 'demons' 'lennon' 'bye' 'dev' 'weller''sasquatch' 'botched' 'xica' 'darkman' 'woo' 'casper' 'doodlebops''smallville' 'wei' 'scanners' 'steve' 'pokemon']
tf-idf 较小的特征要么是在许多文档里都很常用,要么就是很少使用,且仅出现在非常长的文档中。有趣的是,许多 tf-idf 较大的特征实际上对应的是特定的演出或电影。这些术语仅出现在这些特定演出或电影的评论中,但往往在这些评论中多次出现。例如,对于 "pokemon"
、"smallville"
和 "doodlebops"
是显而易见的,但这里的 "scanners"
实际上指的也是电影标题。这些单词不太可能有助于我们的情感分类任务(除非有些电影的评价可能普遍偏正面或偏负面),但肯定包含了关于评论的大量具体信息。
我们还可以找到逆向文档频率较低的单词,即出现次数很多,因此被认为不那么重要的单词。训练集的逆向文档频率值被保存在 idf_
属性中:
sorted_by_idf = np.argsort(vectorizer.idf_)
print("Features with lowest idf:\n{}".format(feature_names[sorted_by_idf[:100]]))
Features with lowest idf: ['the' 'and' 'of' 'to' 'this' 'is' 'it' 'in' 'that' 'but' 'for' 'with''was' 'as' 'on' 'movie' 'not' 'have' 'one' 'be' 'film' 'are' 'you' 'all''at' 'an' 'by' 'so' 'from' 'like' 'who' 'they' 'there' 'if' 'his' 'out''just' 'about' 'he' 'or' 'has' 'what' 'some' 'good' 'can' 'more' 'when''time' 'up' 'very' 'even' 'only' 'no' 'would' 'my' 'see' 'really' 'story''which' 'well' 'had' 'me' 'than' 'much' 'their' 'get' 'were' 'other''been' 'do' 'most' 'don' 'her' 'also' 'into' 'first' 'made' 'how' 'great''because' 'will' 'people' 'make' 'way' 'could' 'we' 'bad' 'after' 'any''too' 'then' 'them' 'she' 'watch' 'think' 'acting' 'movies' 'seen' 'its''him']
正如所料,这些词大多是英语中的停用词,比如 "the"
和 "no"
。但有些单词显然是电影评论特有的,比如 "movie"
、"film"
、"time"
、"story"
等。有趣的是,"good"
、"great"
和 "bad"
也属于频繁出现的单词,因此根据 tf-idf 度量也属于“不太相关”的单词,尽管我们可能认为这些单词对情感分析任务非常重要。
四、研究模型系数
最后,我们详细看一下 Logistic 回归模型从数据中实际学到的内容。由于特征数量非常多(删除出现次数不多的特征之后还有 27 271 个),所以显然我们不能同时查看所有系数。但是,我们可以查看最大的系数,并查看这些系数对应的单词。我们将使用基于 tf-idf 特征训练的最后一个模型。
下面这张条形图(图 7-2)给出了 Logistic 回归模型中最大的 25 个系数与最小的 25 个系数,其高度表示每个系数的大小:
import mglearn
import matplotlib.pyplot as pltmglearn.tools.visualize_coefficients(grid.best_estimator_.named_steps["logisticregression"].coef_,feature_names, n_top_features=40)plt.tight_layout()
plt.savefig('Images/00HandleTextData-01.png', bbox_inches='tight')
plt.show()
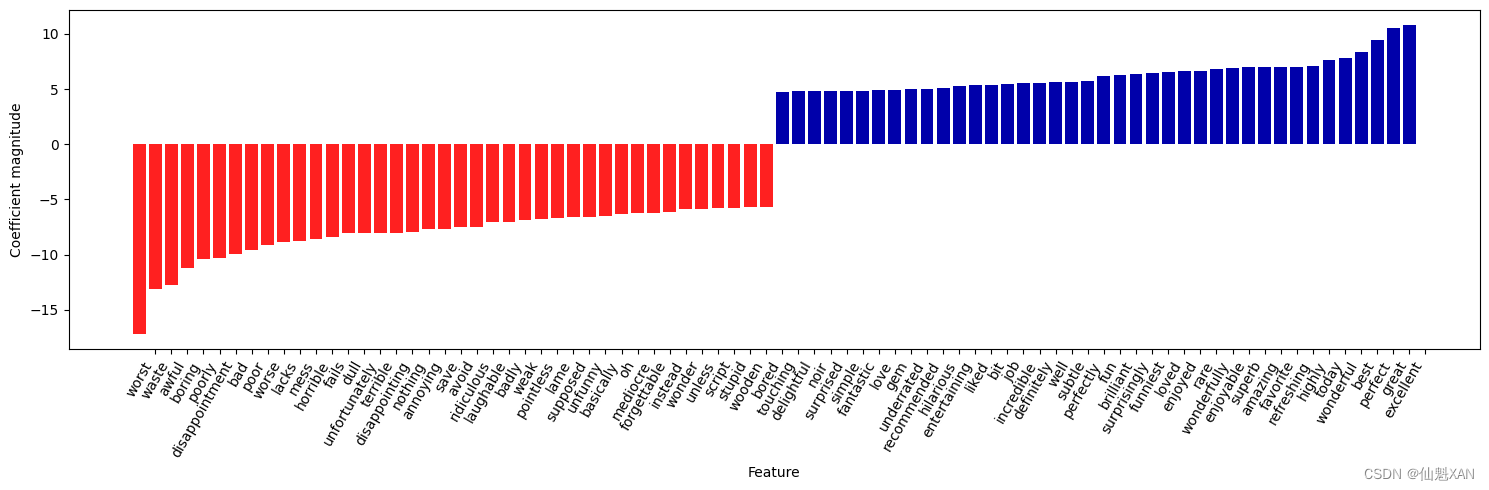
左侧的负系数属于模型找到的表示负面评论的单词,而右侧的正系数属于模型找到的表示正面评论的单词。大多数单词都是非常直观的,比如 "worst"
(最差)、"waste"
(浪费)、"disappointment"
(失望)和 "laughable"
(可笑)都表示不好的电影评论,而 "excellent"
(优秀)、"wonderful"
(精彩)、"enjoyable"
(令人愉悦)和 "refreshing"
(耳目一新)则表示正面的电影评论。有些词的含义不那么明确,比如 "bit"
(一点)、"job"
(工作)和 "today"
(今天),但它们可能是类似 "good job"
(做得不错)和“best today
”(今日最佳)等短语的一部分。
五、多个单词的词袋(n 元分词)
使用词袋表示的主要缺点之一是完全舍弃了单词顺序。因此,“it's bad, not good at all”(电影很差,一点也不好)和“it's good, not bad at all”(电影很好,还不错)这两个字符串的词袋表示完全相同,尽管它们的含义相反。将“not”(不)放在单词前面,这只是上下文很重要的一个例子(可能是一个极端的例子)。幸运的是,使用词袋表示时有一种获取上下文的方法,就是不仅考虑单一词例的计数,而且还考虑相邻的两个或三个词例的计数。
两个词例被称为二元分词 (bigram),三个词例被称为三元分词 (trigram),更一般的词例序列被称为 n 元分词 (n -gram)。我们可以通过改变 CountVectorizer
或 TfidfVectorizer
的 ngram_range
参数来改变作为特征的词例范围。ngram_range
参数是一个元组,包含要考虑的词例序列的最小长度和最大长度。下面是在之前用过的玩具数据上的一个示例:
print("bards_words:\n{}".format(bards_words))
bards_words: ['The fool doth think he is wise,', 'but the wise man knows himself to be a fool']
默认情况下,为每个长度最小为 1 且最大为 1 的词例序列(或者换句话说,刚好 1 个词例)创建一个特征——单个词例也被称为一元分词 (unigram):
cv = CountVectorizer(ngram_range=(1, 1)).fit(bards_words)
print("Vocabulary size: {}".format(len(cv.vocabulary_)))
print("Vocabulary:\n{}".format(cv.get_feature_names_out()))
Vocabulary size: 13 Vocabulary: ['be' 'but' 'doth' 'fool' 'he' 'himself' 'is' 'knows' 'man' 'the' 'think''to' 'wise']
要想仅查看二元分词(即仅查看由两个相邻词例组成的序列),可以将 ngram_range
设置为 (2, 2)
:
cv = CountVectorizer(ngram_range=(2, 2)).fit(bards_words)
print("Vocabulary size: {}".format(len(cv.vocabulary_)))
print("Vocabulary:\n{}".format(cv.get_feature_names_out()))
Vocabulary size: 14 Vocabulary: ['be fool' 'but the' 'doth think' 'fool doth' 'he is' 'himself to''is wise' 'knows himself' 'man knows' 'the fool' 'the wise' 'think he''to be' 'wise man']
使用更长的词例序列通常会得到更多的特征,也会得到更具体的特征。bard_words
的两个短语中没有相同的二元分词:
print("Transformed data (dense):\n{}".format(cv.transform(bards_words).toarray()))
Transformed data (dense): [[0 0 1 1 1 0 1 0 0 1 0 1 0 0][1 1 0 0 0 1 0 1 1 0 1 0 1 1]]
对于大多数应用而言,最小的词例数量应该是 1,因为单个单词通常包含丰富的含义。在大多数情况下,添加二元分词会有所帮助。添加更长的序列(一直到五元分词)也可能有所帮助,但这会导致特征数量的大大增加,也可能会导致过拟合,因为其中包含许多非常具体的特征。原则上来说,二元分词的数量是一元分词数量的平方,三元分词的数量是一元分词数量的三次方,从而导致非常大的特征空间。在实践中,更高的 n 元分词在数据中的出现次数实际上更少,原因在于(英语)语言的结构,不过这个数字仍然很大。
下面是在 bards_words
上使用一元分词、二元分词和三元分词的结果:
cv = CountVectorizer(ngram_range=(1, 3)).fit(bards_words)print("Vocabulary size: {}".format(len(cv.vocabulary_)))
print("Vocabulary:\n{}".format(cv.get_feature_names_out()))
Vocabulary size: 39 Vocabulary: ['be' 'be fool' 'but' 'but the' 'but the wise' 'doth' 'doth think''doth think he' 'fool' 'fool doth' 'fool doth think' 'he' 'he is''he is wise' 'himself' 'himself to' 'himself to be' 'is' 'is wise''knows' 'knows himself' 'knows himself to' 'man' 'man knows''man knows himself' 'the' 'the fool' 'the fool doth' 'the wise''the wise man' 'think' 'think he' 'think he is' 'to' 'to be' 'to be fool''wise' 'wise man' 'wise man knows']
我们在 IMDb 电影评论数据上尝试使用 TfidfVectorizer
,并利用网格搜索找出 n 元分词的最佳设置:
pipe = make_pipeline(TfidfVectorizer(min_df=5), LogisticRegression())
# 运行网格搜索需要很长时间,因为网格相对较大,且包含三元分词
param_grid = {"logisticregression__C": [0.001, 0.01, 0.1, 1, 10, 100],"tfidfvectorizer__ngram_range": [(1, 1), (1, 2), (1, 3)]}grid = GridSearchCV(pipe, param_grid, cv=5)
grid.fit(text_train, y_train)
print("Best cross-validation score: {:.2f}".format(grid.best_score_))
print("Best parameters:\n{}".format(grid.best_params_))
Best cross-validation score: 0.91 Best parameters: {'logisticregression__C': 100, 'tfidfvectorizer__ngram_range': (1, 3)}
从结果中可以看出,我们添加了二元分词特征与三元分词特征之后,性能提高了一个百分点多一点。我们可以将交叉验证精度作为 ngram_range
和 C
参数的函数并用热图可视化,正如我们在之前所做的那样(见图 7-3):
def drawHeatmap(scores, xlabel,ylabel='True label', fmt='{:0.2f}', colorbar=None):# 反转scores数组的行顺序,以便(0, 0)在左下角 scores = scores[::-1, :] # 绘制热图 fig, ax = plt.subplots() if(colorbar is None):colorbar = scorescax = ax.matshow(colorbar, cmap='viridis') fig.colorbar(cax) # 假设param_grid['gamma']和param_grid['C']已经定义 # 这里我们使用字符串列表作为示例 param_grid = { 'gamma': ['0.001','0.01', '0.1', '1', '10', '100'], 'C': ['(1,1)','(1,2)','(1,3)']} # 设置xticks和yticks的位置 ticks_x = np.arange(len(param_grid['gamma'])) ticks_y = np.arange(len(param_grid['C']))[::-1] # 反转yticks的顺序以匹配反转的scores # 设置xticklabels和yticklabels ax.set_xticks(ticks_x) ax.set_xticklabels(param_grid['gamma']) ax.xaxis.set_tick_params(rotation=45) ax.set_yticks(ticks_y) ax.set_yticklabels(param_grid['C']) # 设置x轴和y轴的标签 ax.set_xlabel(xlabel) ax.set_ylabel(ylabel) # 添加数值到每个单元格 for (i, j), z in np.ndenumerate(scores): ax.text(j, i, fmt.format(z), ha='center', va='center') # 从网格搜索中提取分数
scores = grid.cv_results_['mean_test_score'].reshape(-1, 3).T
# 热图可视化
# heatmap = mglearn.tools.heatmap(
# scores, xlabel="C", ylabel="ngram_range", cmap="viridis", fmt="%.3f",
# xticklabels=param_grid['logisticregression__C'],
# yticklabels=param_grid['tfidfvectorizer__ngram_range'])drawHeatmap(scores,xlabel="C", ylabel="ngram_range",fmt='{:0.3f}')plt.tight_layout()
plt.savefig('Images/00HandleTextData-02.png', bbox_inches='tight')
plt.show()
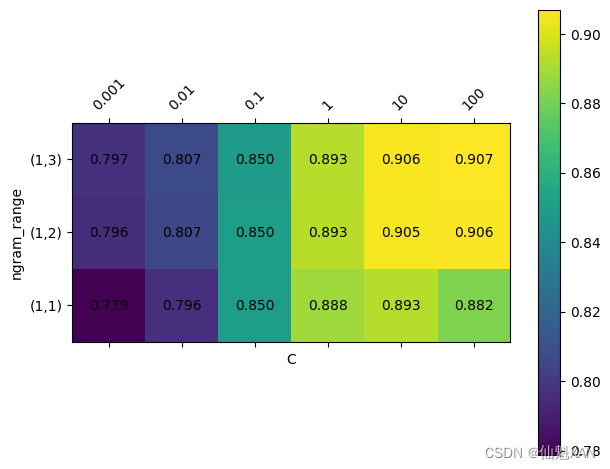
从热图中可以看出,使用二元分词对性能有很大提高,而添加三元分词对精度只有很小贡献。为了更好地理解模型是如何改进的,我们可以将最佳模型的重要系数可视化,其中包含一元分词、二元分词和三元分词(见图 7-4):
# 提取特征名称与系数
vect = grid.best_estimator_.named_steps['tfidfvectorizer']
feature_names = np.array(vect.get_feature_names_out())
coef = grid.best_estimator_.named_steps['logisticregression'].coef_
mglearn.tools.visualize_coefficients(coef, feature_names, n_top_features=40)plt.tight_layout()
plt.savefig('Images/00HandleTextData-03.png', bbox_inches='tight')
plt.show()
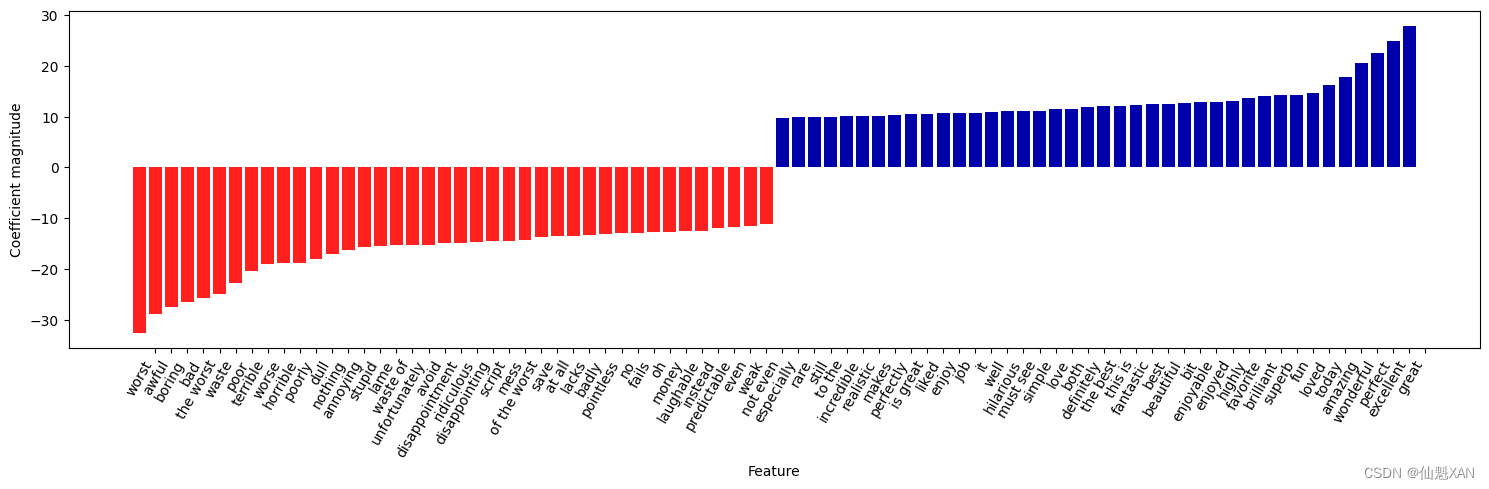
有几个特别有趣的特征,它们包含单词 "worth"
(值得),而这个词本身并没有出现在一元分词模型中:"not worth"
(不值得)表示负面评论,而 "definitely worth"
(绝对值得)和 "well worth"
(很值得)表示正面评论。这是上下文影响 "worth"
一词含义的主要示例。
接下来,我们只将三元分词可视化,以进一步深入了解这些特征有用的原因。许多有用的二元分词和三元分词都由常见的单词组成,这些单词本身可能没有什么信息量,比如 "none of the"
(没有一个)、"the only good"
(唯一好的)、"on and on"
(不停地)、"this is one"
(这是一部)、"of the most"
(最)等短语中的单词。但是,与一元分词特征的重要性相比,这些特征的影响非常有限,正如图 7-5 所示。
# 找到三元分词特征
mask = np.array([len(feature.split(" ")) for feature in feature_names]) == 3
# 仅将三元分词特征可视化
mglearn.tools.visualize_coefficients(coef.ravel()[mask],feature_names[mask], n_top_features=40)plt.tight_layout()
plt.savefig('Images/00HandleTextData-04.png', bbox_inches='tight')
plt.show()
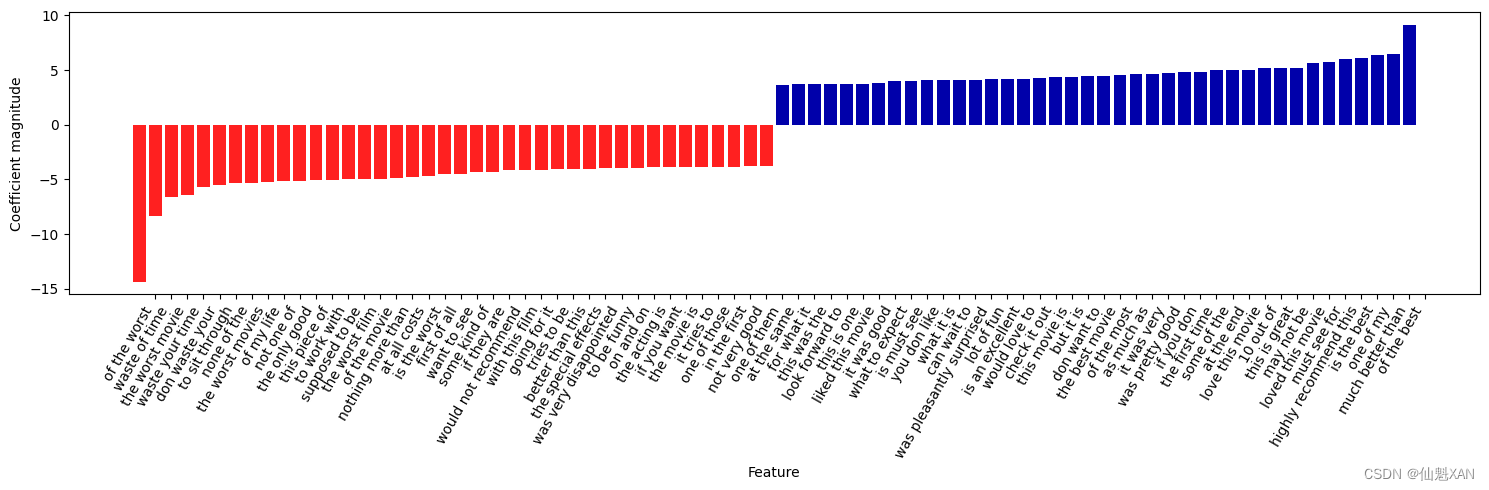
六、高级分词、词干提取与词形还原
如前所述,CountVectorizer
和 TfidfVectorizer
中的特征提取相对简单,还有更为复杂的方法。在更加复杂的文本处理应用中,通常需要改进的步骤是词袋模型的第一步:分词(tokenization)。这一步骤为特征提取定义了一个单词是如何构成的。
我们前面看到,词表中通常同时包含某些单词的单数形式和复数形式,比如 "drawback"
和 "drawbacks"
、"drawer"
和 "drawers"
、"drawing"
和 "drawings"
。对于词袋模型而言,"drawback"
和 "drawbacks"
的语义非常接近,区分二者只会增加过拟合,并导致模型无法充分利用训练数据。同样我们还发现,词表中包含像 "replace"
、"replaced"
、"replace ment"
、"replaces"
和 "replacing"
这样的单词,它们都是动词“to replace”的不同动词形式或相关名词。与名词的单复数形式一样,将不同的动词形式及相关单词视为不同的词例,这不利于构建具有良好泛化性能的模型。
这个问题可以通过用词干 (word stem)表示每个单词来解决,这一方法涉及找出 [ 或合并 (conflate)] 所有具有相同词干的单词。如果使用基于规则的启发法来实现(比如删除常见的后缀),那么通常将其称为词干提取 (stemming)。如果使用的是由已知单词形式组成的字典(明确的且经过人工验证的系统),并且考虑了单词在句子中的作用,那么这个过程被称为词形还原 (lemmatization),单词的标准化形式被称为词元 (lemma)。词干提取和词形还原这两种处理方法都是标准化 (normalization)的形式之一,标准化是指尝试提取一个单词的某种标准形式。标准化的另一个有趣的例子是拼写校正,这种方法在实践中很有用,但超出了本书的范围。
为了更好地理解标准化,我们来对比一种词干提取方法(Porter 词干提取器,一种广泛使用的启发法集合,从 nltk
包导入)与 spacy
包 (安装 spacy
包之后需要下载相应的语言包,你可以在命令行输入 python3 -m spacy download en
来下载英语语言包) 中实现的词形还原(想了解接口的细节,请参阅 nltk
(NLTK :: Natural Language Toolkit )和 spacy
(spaCy · Industrial-strength Natural Language Processing in Python )的文档。我们这里更关注一般性原则):
import spacy
import nltk# 加载spacy的英语模型
en_nlp = spacy.load('en_core_web_sm')
# 将nltk的Porter词干提取器实例化
stemmer = nltk.stem.PorterStemmer()# 定义一个函数来对比spacy中的词形还原与nltk中的词干提取
def compare_normalization(doc):# 在spacy中对文档进行分词doc_spacy = en_nlp(doc)# 打印出spacy找到的词元print("Lemmatization:")print([token.lemma_ for token in doc_spacy])# 打印出Porter词干提取器找到的词例print("Stemming:")print([stemmer.stem(token.norm_.lower()) for token in doc_spacy])
我们将用一个句子来比较词形还原与 Porter 词干提取器,以显示二者的一些区别(1.7.5 版的 spacy
将 'our'
、'i'
等代词全部还原为 '-PRON-'
,详情请参见 spacy
官方文档):
compare_normalization(u"Our meeting today was worse than yesterday, ""I'm scared of meeting the clients tomorrow.")
Lemmatization: ['our', 'meeting', 'today', 'be', 'bad', 'than', 'yesterday', ',', 'I', 'be', 'scared', 'of', 'meet', 'the', 'client', 'tomorrow', '.'] Stemming: ['our', 'meet', 'today', 'wa', 'wors', 'than', 'yesterday', ',', 'i', 'am', 'scare', 'of', 'meet', 'the', 'client', 'tomorrow', '.']
词干提取总是局限于将单词简化成词干,因此 "was"
变成了 "wa"
,而词形还原可以得到正确的动词基本词形 "be"
。同样,词形还原可以将 "worse"
标准化为 "bad"
,而词干提取得到的是 "wors"
。另一个主要区别在于,词干提取将两处 "meeting"
都简化为 "meet"
。利用词形还原,第一处 "meeting"
被认为是名词,所以没有变化,而第二处 "meeting"
被认为是动词,所以变为 "meet"
。一般来说,词形还原是一个比词干提取更复杂的过程,但用于机器学习的词例标准化时通常可以给出比词干提取更好的结果。
虽然 scikit-learn
没有实现这两种形式的标准化,但 CountVectorizer
允许使用 tokenizer
参数来指定使用你自己的分词器将每个文档转换为词例列表。我们可以使用 spacy
的词形还原了创建一个可调用对象,它接受一个字符串并生成一个词元列表:
# 技术细节:我们希望使用由CountVectorizer所使用的基于正则表达式的分词器,
# 并仅使用spacy的词形还原。
# 为此,我们将en_nlp.tokenizer(spacy分词器)替换为基于正则表达式的分词。
# 引入 CountVectorizer
from sklearn.feature_extraction.text import CountVectorizer
import re
# 在CountVectorizer中使用的正则表达式
regexp = re.compile('(?u)\\b\\w\\w+\\b')# 加载spacy语言模型,并保存旧的分词器
en_nlp = spacy.load('en_core_web_sm')
old_tokenizer = en_nlp.tokenizer
# 将分词器替换为前面的正则表达式
en_nlp.tokenizer = lambda string: old_tokenizer.tokens_from_list(regexp.findall(string))# 用spacy文档处理管道创建一个自定义分词器
# (现在使用我们自己的分词器)
def custom_tokenizer(document):doc_spacy = en_nlp(document, entity=False, parse=False)return [token.lemma_ for token in doc_spacy]# 利用自定义分词器来定义一个计数向量器
lemma_vect = CountVectorizer(tokenizer=custom_tokenizer, min_df=5)
我们变换数据并检查词表的大小:
text_train = reviews_train.data
# 利用带词形还原的CountVectorizer对text_train进行变换
X_train_lemma = lemma_vect.fit_transform(text_train)
print("X_train_lemma.shape: {}".format(X_train_lemma.shape))# 标准的CountVectorizer,以供参考
vect = CountVectorizer(min_df=5).fit(text_train)
X_train = vect.transform(text_train)
print("X_train.shape: {}".format(X_train.shape))
X_train_lemma.shape: (25000, 91079) X_train.shape: (25000, 27272)
从输出中可以看出,词形还原将特征数量从 27 271 个(标准的 CountVectorizer
处理过程)减少到 21 596 个。词形还原可以被看作是一种正则化,因为它合并了某些特征。因此我们预计,数据集很小时词形还原对性能的提升最大。为了说明词形还原的作用,我们将使用 StratifiedShuffleSplit
做交叉验证,仅使用 1% 的数据作为训练数据,其余数据作为测试数据:
# 仅使用1%的数据作为训练集来构建网格搜索
from sklearn.model_selection import StratifiedShuffleSplitparam_grid = {'C': [0.001, 0.01, 0.1, 1, 10]}
cv = StratifiedShuffleSplit(n_splits=5, test_size=0.99,train_size=0.01, random_state=0)
grid = GridSearchCV(LogisticRegression(), param_grid, cv=cv)
# 利用标准的CountVectorizer进行网格搜索
grid.fit(X_train, y_train)
print("Best cross-validation score ""(standard CountVectorizer): {:.3f}".format(grid.best_score_))
# 利用词形还原进行网格搜索
grid.fit(X_train_lemma, y_train)
print("Best cross-validation score ""(lemmatization): {:.3f}".format(grid.best_score_))
Best cross-validation score (standard CountVectorizer): 0.717 Best cross-validation score (lemmatization): 0.743
在这个例子中,词形还原对性能有较小的提高。与许多特征提取技术一样,其结果因数据集的不同而不同。词形还原与词干提取有时有助于构建更好的模型(或至少是更简洁的模型),所以我们建议你,在特定任务中努力提升最后一点性能时可以尝试下这些技术。
七、主题建模与文档聚类
常用于文本数据的一种特殊技术是主题建模 (topic modeling),这是描述将每个文档分配给一个或多个主题的任务(通常是无监督的)的概括性术语。这方面一个很好的例子是新闻数据,它们可以被分为“政治”“体育”“金融”等主题。如果为每个文档分配一个主题,那么这是一个文档聚类任务。如果每个文档可以有多个主题,那么这个任务与之前的分解方法有关。我们学到的每个成分对应于一个主题,文档表示中的成分系数告诉们这个文档与该主题的相关性强弱。通常来说,人们在谈论主题建模时,他们指的是一种叫作隐含狄利克雷分布 (Latent Dirichlet Allocation,LDA)的特定分解方法。
1、隐含狄利克雷分布
从直观上来看,LDA 模型试图找出频繁共同出现的单词群组(即主题)。LDA 还要求,每个文档可以被理解为主题子集的“混合”。重要的是要理解,机器学习模型所谓的“主题”可能不是我们通常在日常对话中所说的主题,而是更类似于 PCA 或 NMF 所提取的成分,它可能具有语义,也可能没有。即使 LDA“主题”具有语义,它可能也不是我们通常所说的主题。回到新闻文章的例子,我们可能有许多关于体育、政治和金融的文章,由两位作者所写。在一篇政治文章中,我们预计可能会看到“州长”“投票”“党派”等词语,而在一篇体育文章中,我们预计可能会看到类似“队伍”“得分”和“赛季”之类的词语。这两组词语可能会同时出现,而例如“队伍”和“州长”就不太可能同时出现。但是,这并不是我们预计可能同时出现的唯一的单词群组。这两位记者可能偏爱不同的短语或者选择不同的单词。可能其中一人喜欢使用“划界”(demarcate)这个词,而另一人喜欢使用“两极分化”(polarize)这个词。其他“主题”可能是“记者 A 常用的词语”和“记者 B 常用的词语”,虽然这并不是通常意义上的主题。
我们将 LDA 应用于电影评论数据集,来看一下它在实践中的效果。对于无监督的文本文档模型,通常最好删除非常常见的单词,否则它们可能会支配分析过程。我们将删除至少在 15% 的文档中出现过的单词,并在删除前 15% 之后,将词袋模型限定为最常见的 10 000 个单词(还有另一种机器学习模型通常也简称为 LDA,那就是线性判别分析(Linear Discriminant Analysis),一种线性分类模型。这造成了很多混乱。在本书中,LDA 是指隐含狄利克雷分布。):
vect = CountVectorizer(max_features=10000, max_df=.15)
X = vect.fit_transform(text_train)
我们将学习一个包含 10 个主题的主题模型,它包含的主题个数很少,我们可以查看所有主题。与 NMF 中的分量类似,主题没有内在的顺序,而改变主题数量将会改变所有主题(事实上,NMF 和 LDA 解决的是非常相关的问题,我们也可以用 NMF 来提取主题)。 我们将使用 "batch"
学习方法,它比默认方法("online"
)稍慢,但通常会给出更好的结果。我们还将增大 max_iter
,这样会得到更好的模型:
from sklearn.decomposition import LatentDirichletAllocation
# 创建 LDA 模型实例
lda = LatentDirichletAllocation(n_components=10, learning_method="batch",max_iter=25, random_state=0)
# 我们在一个步骤中构建模型并变换数据
# 计算变换需要花点时间,二者同时进行可以节省时间
document_topics = lda.fit_transform(X)
与之前中所讲的分解方法类似,LatentDirichletAllocation
有一个 components_
属性,其中保存了每个单词对每个主题的重要性。components_
的大小为 (n_topics, n_words)
:
lda.components_.shape
(10, 10000)
为了更好地理解不同主题的含义,我们将查看每个主题中最重要的单词。print_topics
函数为这些特征提供了良好的格式:
# 对于每个主题(components_的一行),将特征排序(升序)
# 用[:, ::-1]将行反转,使排序变为降序
sorting = np.argsort(lda.components_, axis=1)[:, ::-1]
# 从向量器中获取特征名称
feature_names = np.array(vect.get_feature_names_out())# 打印出前10个主题:
mglearn.tools.print_topics(topics=range(10), feature_names=feature_names,sorting=sorting, topics_per_chunk=5, n_words=10)
topic 0 topic 1 topic 2 topic 3 topic 4 -------- -------- -------- -------- -------- director show book family funny work series original young comedy performance war 10 father cast actors episode now us role cast tv again woman humor screen years world own fun performances american saw world jokes role episodes read real actors both world didn mother performance quite shows am between always topic 5 topic 6 topic 7 topic 8 topic 9 -------- -------- -------- -------- -------- horror music original thing action gore john team worst police effects old series didn murder blood young jack nothing killer pretty girl action minutes crime budget song new guy plays house gets down actually lee zombie dance tarzan want gets dead songs freddy going role low rock indian re cop
从重要的单词来看,主题 1 似乎是关于历史和战争的电影,主题 2 可能是关于糟糕的喜剧,主题 3 可能是关于电视连续剧,主题 4 可能提取了一些非常常见的单词,而主题 6 似乎是关于儿童电影,主题 8 似乎提取了与获奖相关的评论。仅使用 10 个主题,每个主题都需要非常宽泛,才能共同涵盖我们的数据集中所有不同类型的评论。
接下来,我们将学习另一个模型,这次包含 100 个主题。使用更多的主题,将使得分析过程更加困难,但更可能使主题专门针对于某个有趣的数据子集:
lda100 = LatentDirichletAllocation(n_components=100, learning_method="batch",max_iter=25, random_state=0)
document_topics100 = lda100.fit_transform(X)
查看所有 100 个主题可能有点困难,所以我们选取了一些有趣的而且有代表性的主题:
topics = np.array([7, 16, 24, 25, 28, 36, 37, 45, 51, 53, 54, 63, 89, 97])sorting = np.argsort(lda100.components_, axis=1)[:, ::-1]
feature_names = np.array(vect.get_feature_names_out())
mglearn.tools.print_topics(topics=topics, feature_names=feature_names,sorting=sorting, topics_per_chunk=7, n_words=20)
topic 7 topic 16 topic 24 topic 25 topic 28 topic 36 topic 37 -------- -------- -------- -------- -------- -------- -------- drew didn ben emma years role ship baseball going lines elvira saw oscar titanic maria seemed actors jeremy again award crew sports things director hudson remember actor day whale doesn audience paltrow ago picture world ben interesting every sirk now won air red nothing worst austen watched roles sea angels bit without bacall old supporting lost barrymore lot simply mr am academy plane pitch felt dialogue kyle since nominated macarthur fever thing parody malone before performance events frankie seems us thompson last grant real sox ending script stack didn winning death team might self dorothy few cast war irish work less preston came actors earth wendigo feel care wind year year captain deaf though attempt robert day years disaster casper actually mind written thought century james deer thought shallow jane found three water gypo quite imagine toni find comedy london topic 45 topic 51 topic 53 topic 54 topic 63 topic 89 topic 97 -------- -------- -------- -------- -------- -------- -------- documentary world moon show drugs jack our footage green mike shows drug king us festival new columbo tv freeman hotel human interviews bus cat television money stephen world documentaries heston hat episode disturbing kubrick own media streets mel season real shining reality interesting panic mst3k episodes experiment nicholson every stooges city brooks always ll danny real subject orleans cassidy air morgan stanley mind history health austin funny abuse wendy need chavez palance hyde program rat wang understand wrestling plague baldwin reality rats zombi feel scorsese 3d skull every spacey gere want shorts director tall lot less room things making macy ho new 10 flock different verhoeven kazan myers abc justin claire nature gerard around cure seasons nothing bruno lives christine mario gackt now kevin birds without international soylent full sitcom addicted snow soul narration big ken real timberlake find makes
这次我们提取的主题似乎更加具体,不过很多都难以解读。主题 7 似乎是关于恐怖电影和惊悚片,主题 16 和 54 似乎是关于不好的评论,而主题 63 似乎主要是关于喜剧的正面评论。如果想要利用发现的主题做出进一步的推断,那么我们应该查看分配给这些主题的文档,以验证我们通过查看每个主题排名最靠前的单词所得到的直觉。例如,主题 45 似乎是关于音乐的。我们来查看哪些评论被分配给了这个主题:
# 按主题45“music”进行排序
music = np.argsort(document_topics100[:, 45])[::-1]
# 打印出这个主题最重要的前5个文档
for i in music[:10]:# 显示前两个句子print(b".".join(text_train[i].split(b".")[:2]) + b".\n")
b"This movie is basically a documentary of the chronologically ordered series of events that took place from April 10, 2002 through April 14, 2002 in the Venezuelan Presidential Palace, Caracas Venezuela.<br /><br />The pathos of the movie is real and one feels the pain, sorrow and joy of the people who lived through this failed coup d'etat of President Hugo Chavez.\n" b'Reviewed at the World Premiere screening Sept. 9, 2006 at the Isabel Bader Theatre during the Toronto International Film Festival (TIFF).\n' b'Moe and Larry are newly henpecked husbands, having married Shemp\'s demanding sisters. At his music studio, Shemp learns he will inherit a fortune if he marries someone himself! <br /><br />"Husbands Beware" is a remake of 1947\'s "Brideless Groom," widely considered by many to be one of the best Stooge films with Shemp.\n' b'Sharp, well-made documentary focusing on Mardi Gras beads. I have always liked this approach to film-making - communicate ideas about a larger, more complex, and often inscrutable phenomenon by breaking the issue down into something familiar and close to home.\n' b"This is a great movie, it shows what our government will to to other countries if we don't like their government. This isn't as bad as what Reagan and Bush number one did to South America, but the US still has no business messing around with other countries like this.\n" b'Another good Stooge short!Christine McIntyre is so lovely and evil and the same time in this one!She is such a great actress!The Stooges are very good and especially Shemp and Larry!This to is a good one to watch around Autumn time!.\n' b'"Why did they make them so big? Why didn\'t they just give the money to the poor?" The question about cathedrals was asked by a student to Mr. Harvey during a school field trip to Salisbury Cathedral.\n' b'In Micro Phonies the stooges are at there best. In this short the trio are handymen working in a recording studio.\n' b'This documentary has been aired on both RTE and BBC in the last number of months. Having seen it twice now I would recommend it to anyone with an interest in media and documentary film making.\n' b'This three stooges flick is at a tie with my other favorite flick "Disorder in the Court". This is an uproar of laughter for any Three Stooges fan to enjoy.\n'
可以看出,这个主题涵盖许多以音乐为主的评论,从音乐剧到传记电影,再到最后一条评论中难以归类的类型。查看主题还有一种有趣的方法,就是通过对所有评论的 document_topics
进行求和来查看每个主题所获得的整体权重。我们用最常见的两个单词为每个主题命名。图 7-6 给出了学到的主题权重:
fig, ax = plt.subplots(1, 2, figsize=(10, 10))
topic_names = ["{:>2} ".format(i) + " ".join(words)for i, words in enumerate(feature_names[sorting[:, :2]])]
# 两列的条形图:
for col in [0, 1]:start = col * 50end = (col + 1) * 50ax[col].barh(np.arange(50), np.sum(document_topics100, axis=0)[start:end])ax[col].set_yticks(np.arange(50))ax[col].set_yticklabels(topic_names[start:end], ha="left", va="top")ax[col].invert_yaxis()ax[col].set_xlim(0, 2000)yax = ax[col].get_yaxis()yax.set_tick_params(pad=130)plt.tight_layout()
plt.savefig('Images/00HandleTextData-05.png', bbox_inches='tight')
plt.show()
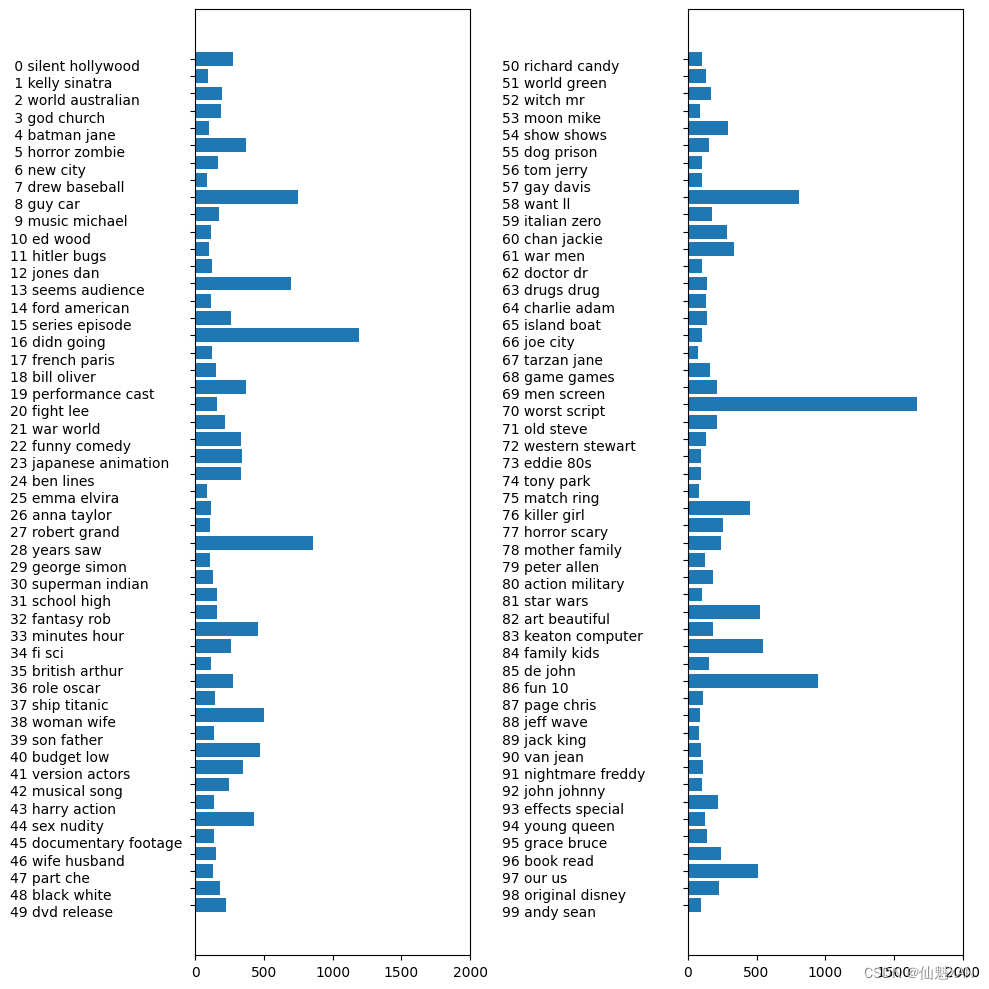
最重要的主题是主题 97,它可能主要包含停用词,可能还有一些稍负面的单词;主题 16 明显是有关负面评论的;然后是一些特定类型的主题与主题 36 和 37,这二者似乎都包含表示赞美的单词。
除了几个不太具体的主题之外,LDA 似乎主要发现了两种主题:特定类型的主题与特定评分的主题。这是一个有趣的发现,因为大部分评论都由一些与电影相关的评论与一些证明或强调评分的评论组成。
在没有标签的情况下(或者像本章的例子这样,即使有标签的情况下),像 LDA 这样的主题模型是理解大型文本语料库的有趣方法。不过 LDA 算法是随机的,改变 random_state
参数可能会得到完全不同的结果。虽然找到主题可能很有用,但对于从无监督模型中得出的任何结论都应该持保留态度,我们建议通过查看特定主题中的文档来验证你的直觉。 LDA.transform
方法生成的主题有时也可以用于监督学习的紧凑表示。当训练样例很少时,这一方法特别有用。
附录:
一、参考文献
参考文献:[德] Andreas C. Müller [美] Sarah Guido 《Python Machine Learning Basics Tutorial》
二、spacy 环境配置
1、spacy 如何安装,以及数据集使用,官网介绍如下
https://github.com/explosion/spacy-models/releases
进入后,可以看到如下界面,并下载支持对应spacy版本的Spacy-models;
此处的 lg 为large的缩写,根据spacy官方文档的解读,
模型指示符 如英文模型en,
后缀为 sm:en_core_web_sm-3.7.1 代表 small 模型;
后缀为 md: 代表 middle 模型;
后缀为 lg: 代表 large 模型;
后缀为 trf: 代表涵盖 transformer 模型;
2、选择需要的模型并找到适合的版本号下载
建议直接按照如下方式 下载到本地(放到python解释器安装的目录,经测试这样的下载速度较快。
查看python解释器安装目录指令:
python -c "import sys; print(sys.executable)"
3、这里以en_core_web_sm-3.5.0 为例
en_core_web_sm-3.5.0地址:Release en_core_web_sm-3.5.0 · explosion/spacy-models · GitHub
首先安装 spacy ,pip install spacy
然后 下载 en_core_web_sm 数据,python -m spacy download en_core_web_sm
如果下载 en_core_web_sm 数据,报如下类似的错:
requests.exceptions.SSLError: HTTPSConnectionPool(host='raw.githubusercontent.com', port=443): Max retries exceeded with url: /explosion/spacy-models/master/compatib ility.json (Caused by SSLError(SSLCertVerificationError(1, '[SSL: CERTIFICATE_VERIFY_FAILED] certificate verify failed: unable to get local issuer certificate (_ssl. c:992)')))
4、下载 en_core_web_sm-3.5.0-py3-none-any.whl
5、可以在安装了spacy 的环境中,安装下载的 en_core_web_sm-3.5.0-py3-none-any.whl
pip install en_core_web_sm-3.5.0-py3-none-any.whl
6、使用下面代码,运行检验即可
import spacynlp = spacy.load("en_core_web_lg")
如果上两行代码运行都未报错,即表明安装spacy成功,模型可以正常调用。