在session上下文中,捕获用户的搜索意图,是一件较为复杂和困难的事情。
一起看一下人大的这篇论文 Large Language Models Know Your Contextual Search Intent: A Prompting Framework for Conversational Search
会话中的搜索意图和query改写
人大的论文中提出了一个简单而有效的提示框架,称为LLM4CS,以利用LLM作为搜索意图解释器来促进会话搜索。具体来说,我们首先提示LLM在多个视角下生成较短的查询重写和较长的假设响应,然后将这些生成的内容聚合成一个集成的表示,以稳健地表示用户的真实搜索意图。在我们的框架下,我们提出三种具体的提示方法和聚合方法,并进行广泛的评估三个广泛使用的会话搜索基准,包括CAsT-19(道尔顿等人,2020),CAsT-20(道尔顿等人,2021),和CAsT-21(道尔顿等人,2022)),全面调查llm对话搜索的有效性。
论文核心内容
论文中吗,主要解决的是从上下文的会话信息中,提取信息,已补充和改写最新的query。解决上下文的关联问题。
论文中提到了三种重写的方式,如下图所示。
- 第一种REW是直接把历史的qa和当前query一起送给模型,然后让模型给出重写后的query。
- 第二种RTR是把历史的qa和当前query一起送给模型,然后让模型给出重写后的query。并给出一个回答。把回答的内容和和重写后的query一起构造成新的query。这里论文中虽然没有提到,但是在谷歌和微软的相关论文中,由于生成的答案通常比重写后的query要长,索引要把重写后的query重复拼接5次,然后再拼接上回答的内容,最后构成最终的query。
- 第三种RAR是把历史的qa和当前query一起送给模型,然后让模型给出重写后的query。并给出一个回答。这里可以生成多个问题和多个回答。也就是可以把复杂的问题进行拆解。
额外地生成假设的响应和正确地聚合多个生成的结果对于提高搜索性能是至关重要的!
论文中提及的query重写方法如何使用?
最简单的方法是,将会话中的每一个qa对都当做内容,和当前的query一起送给LLM。并给LLM一个提示,让LLM根据这些内容去重写query。
Prompt指令如下,让LLM知道是从上下文中,获取信息。
For an information-seeking dialog, please help reformulate the question into rewrite that
can fully express the user‘s information needs without the need of context, but also
generate an informative response to answer the question.
给LLM几个重写的示例
示例中包含了query,和重写的原因,以及重写后的query,最后是LLM针对query的一个简短的回答。
I will give you several example multi-turn dialogs, where each turn contains a question, a
response, and a rewrite. The rewrite part begins with a sentence explaining the reason for
the rewrite.
Example #1:
Question: What should I consider when buying a phone?
Rewrite: This is the first turn. So the question should be rewritten as: What should I
consider when buying a phone?
Response: The design of the phone and the overall …
Question: Cool. Which one would you recommend?
Rewrite: Based on Turn 1, you are inquiring about what should be considered when
buying a phone. So the question should be rewritten as: Cool. Which smartphone would
you recommend for me?
Response: Just because a phone has everything…
…
Example #2:
输入如下:
Your Task (only questions and responses are given):
Context:
Question: What was the basis of the Watergate scandal?
Response: ...
Question: …
Response: …
…
Current Question: So what happened to Nixon?
Rewrite: So what happened to Nixon after the events of the Watergate scandal?
(Now, you should give me the rewrite and an informative response of the **Current
Question** based on the **Context**. The output format should always be: Rewrite:
$Reason. So the question should be rewritten as: $Rewrite\nResponse: $Response. Go
ahead!)
模型输入如下
Rewrite: Based on all previous turns, Nixon was badly involved in the Watergate scandal.
So the question should be rewritten as: So what happened to Nixon after the events of the
Watergate scandal?
Response: With the mounting evidence and loss…
会话数据
思维链
意图聚合
效果如何
首先,RAR和RTR提示方法的性能明显优于REW提示,这表明生成的假设响应可以有效地补充较短的查询重写,从而提高检索性能。然而,即使是与现有基线相比,即使是简单的REW提示也可以实现相当有竞争力的性能,特别是在更具挑战性的CAsT-20和CAsT-21数据集上,它显示了显著的优势(例如,CAsT-20上0.380vs.0.350,CAsT-21上0.465vs.0.385)。这些积极的结果进一步突出了利用LLM进行对话搜索的显著优势。
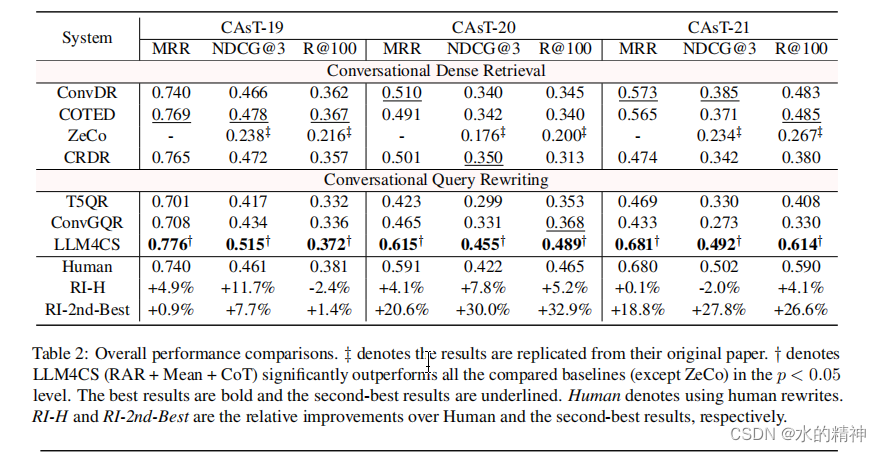
其次,在聚合方法方面,Mean和SC始终优于MaxProb。这些结果表明,仅仅依赖于语言模型的顶级预测可能不能提供足够的可靠性。相反,利用多个结果的集体强度被证明是一个更好的选择。此外,我们观察到,平均聚合方法,它将所有生成的内容融合到最终的搜索意图向量中(公式11),并不始终优于SC(例如,在CAsT-20上),它实际上只融合了一个重写和一个响应。
再看一下思维链CoT对结果的影响
可以明显看到,不管是在哪种意图聚合方式下,启用思维链,几乎总是有正向的效果。