一、说明
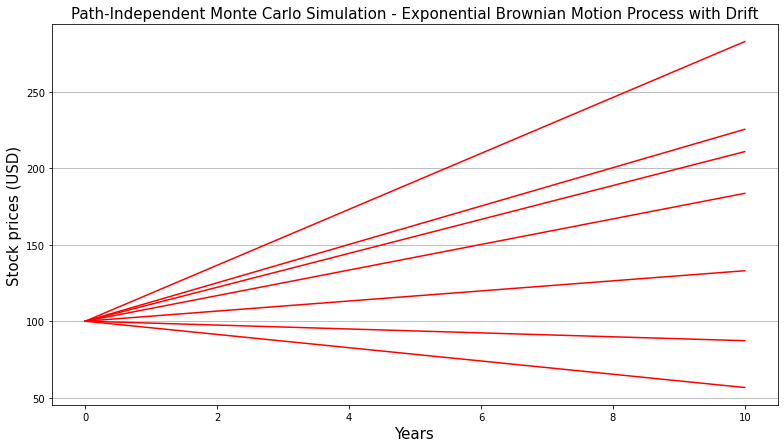
二、赋予动机
为了预测股票的未知未来价值,我将在本文中展示一个与路径无关的 Python 蒙特卡罗模拟,以使用称为指数布朗运动(随机游走)过程的随机过程来模拟股票价格的未来分布。
预测是预测未来的行为,无论是基于历史数据还是在没有历史存在的情况下对未来的猜测。当存在历史数据时,定量或统计方法是最好的,但如果不存在历史数据,那么定性或判断性方法通常是唯一的手段。
三、使用随机过程进行预测的基础知识
随机过程只不过是一个数学定义的方程,可以随着时间的推移产生一系列结果,这些结果本质上不是确定性的。也就是说,它不遵循任何简单的可识别规则,例如价格每年将增加 X 个百分点,或者收入将增加 X 加 Y 个百分点。
根据定义,随机过程是不确定的,可以将数字代入随机过程方程中,每次都获得不同的结果。例如,股票价格的路径本质上是随机的,人们无法可靠地预测确切的股票价格路径。
然而,价格随时间的演变被包裹在产生这些价格的过程中。这个过程是固定的和预先确定的,但结果不是。
因此,通过随机模拟,我们创建了多个价格路径,获得了这些模拟的统计样本,并根据用于生成时间序列的随机过程的性质和参数,对实际价格可能采取的潜在路径进行推断。
四、随机游走:布朗运动
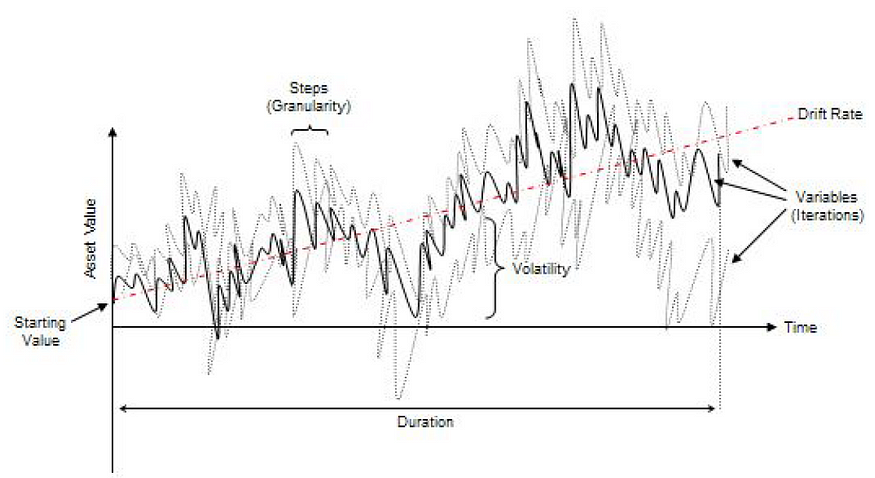
假设一个过程 X,其中
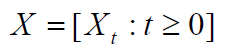
当且仅当

是连续的,且起点是
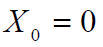
其中 X 呈正态分布,均值为零,方差为 1 或
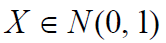
并且时间上的每个增量都依赖于每个先前的增量,并且本身正态分布,均值零和方差 t,使得

然后,过程

遵循指数布朗运动,其中α是漂移参数,σ波动性度量,并且
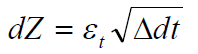
这样
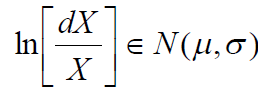
或 X 和 dX 对数呈正态分布。
如果在时间零,
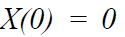
则过程 X 在任何时候的期望值 t 是这样的
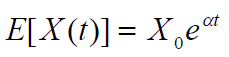
过程 X 在任何时候的方差 t 为
在α存在漂移参数的连续情况下,预期值变为

五、指数布朗运动(随机游走)过程
未知未来值的路径无关指数布朗运动(随机游走)过程采用以下形式
for regular options simulation, or a more generic version takes the form of
where
S0 = the variable’s today value (a.k.a starting value).
T = the forecast horizon (years).
ST = the variable’s future value T years from today (a.k.a final value).
μ = 年化增长率或漂移率。
σ = 年化波动率。
ε = 来自标准化正态分布 Φ(0, 1) 的随机抽取。
为了从一组时间序列数据中估计参数,漂移率μ为相对回报的平均值
而σ是所有
值。
六、蒙特卡罗模拟
蒙特卡罗模拟通常用于没有分析解且其他方法(如二项式树)不太合适的情况。
当用于预测股票的未来价值时,蒙特卡罗模拟使用真实世界的估值结果。现实世界的估值意味着股票的平均增长率必须高于无风险资产。因此,现实世界估值下的漂移率是股票的预期回报率,而风险中性估值/世界下的漂移率是无风险率。
我们对路径进行采样,以获得现实世界中的预期未来价值。考虑今天值为 S0 的股票 S,我们希望从今天起预测其未来价值 T 年。假设预期收益和波动率是恒定的,我们可以预测从今天起股票T年的未来价值如下:
- 在现实世界中对 S 的随机路径(也称为迭代)进行采样。
- 计算股票的未来价值。
- 重复步骤 1 和 2 以获取现实世界中股票未来价值的许多样本值。
- 计算样本未来值的平均值,以获得股票预期未来值的估计值。
假设现实世界中股票所遵循的未知未来价值的过程是
七、Python中的指数布朗运动
考虑一只起始值为 100、漂移率为 5%、年化波动率为 25% 和预测期限为 10 年的股票。让我们根据这些参数和称为指数布朗运动(随机游走)和漂移的随机过程来预测 10 年后的股票未来价值。
由于我们希望运行与路径无关的模拟,因此我们将仅使用一个时间步长。最后,我们将在计算中使用 50,000 次迭代。
因此,S0 = 100,T = 10.0,μ = 0.05,σ = 0.25,N=1,M = 50,000
首先,让我们加载相关的 python 库用于数据科学。
import pandas as pd import numpy as np from matplotlib import pyplot as plt from numpy import random as rn from scipy import stats import scipy.stats as si import seaborn as sns G = 1629562571 np.random.seed(G) from datetime import datetime # datetime object containing current date and time now = datetime.now() dt_string = now.strftime("%d/%m/%Y %H:%M:%S")
其次,让我们设置模型的参数。
S0 = 100 T = 10.0 μ = 0.05 σ = 0.25 M = 50000 N = 1 N
1
第三,让我们为未来的值和随机绘图构建指标
ε = rn.randn(M,N) S = S0*np.ones((M,N+1)) dt = T/N dt
5
第四,让我们构建模型
start_time = datetime.now() for i in range(0,N):S[:,i+1] = S[:,i]*np.exp( (μ-0.5*σ**2)*T + σ*ε[:,i]*np.sqrt(T) )
第五,让我们可视化 8 条路径的模型结果
plt.figure(figsize=(13,7)) fontsize=15 plt.title('Path-Independent Monte Carlo Simulation - Exponential Brownian Motion with Drift',fontsize=fontsize) plt.xlabel('Years',fontsize=fontsize) plt.ylabel('Stock prices (USD)',fontsize=fontsize) plt.grid(axis='y') a = [ rn.randint(0,M) for j in range(1,8)] for runer in a:plt.plot(np.arange(0,T+dt,dt), S[runer], 'red')
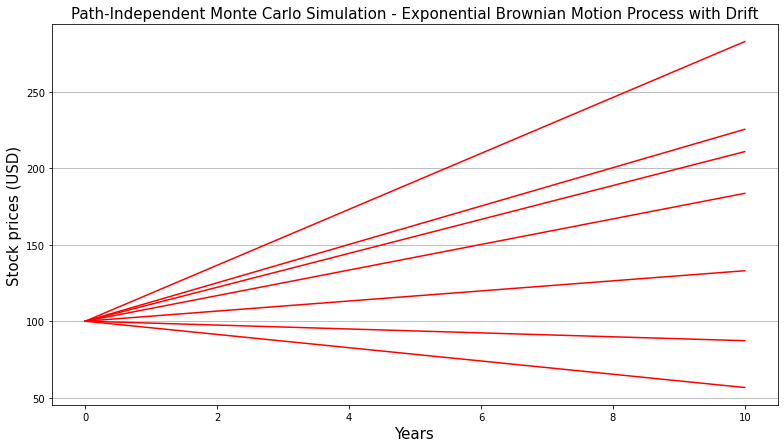
V = (S[:,-1]) print("\033[1m The estimate of the future value of the stock is ${:.2f}".format(np.mean(V))) print("\033[1m The accuracy of the estimate of the future value of the stock is ${:.2f}".format((np.std(V)/np.sqrt(M))))
股票未来价值的估计为 164.78
美元 股票未来价值估计的准确率为 0.69 美元
第七,让我们打印内在值属性输出
time_elapsed = datetime.now() - start_time def NORMSINV(x):x = si.norm.ppf(x)return (x) Workbook_Name = "Exponential Brownian Motion with Drift.ipynb" Number_of_Steps = "{:,.0f}".format(N) Number_of_Iterations = "{:,.0f}".format(M) Number_of_Inputs = "{:,.0f}".format(6) Number_of_Outputs = 1 Sampling_Type = "Latin Hypercube" Simulation_Start_Time = dt_string Simulation_Duration = '{}'.format(time_elapsed) Random_N_Generator = 'Mersenne Twister' e = ['Workbook Name','Number of Steps','Number of Iterations','Number of Inputs','Number of Outputs','Sampling Type',\'Simulation Start Time','Simulation Duration','Random # Generator'] f = [Workbook_Name, Number_of_Steps, Number_of_Iterations, Number_of_Inputs, Number_of_Outputs, Sampling_Type,\Simulation_Start_Time, Simulation_Duration, Random_N_Generator] Per5 = "{:,.1f}".format(np.percentile(V, 5)) P5 = "{:.0%}".format(0.05) Per10 = "{:,.1f}".format(np.percentile(V, 10)) P10 = "{:.0%}".format(0.10) Per15 = "{:,.1f}".format(np.percentile(V, 15)) P15 = "{:.0%}".format(0.15) Per20 = "{:,.1f}".format(np.percentile(V, 20)) P20 = "{:.0%}".format(0.20) Per25 = "{:,.1f}".format(np.percentile(V, 25)) P25 = "{:.0%}".format(0.25) Per30 = "{:,.1f}".format(np.percentile(V, 30)) P30 = "{:.0%}".format(0.30) Per35 = "{:,.1f}".format(np.percentile(V, 35)) P35 = "{:.0%}".format(0.35) Per40 = "{:,.1f}".format(np.percentile(V, 40)) P40 = "{:.0%}".format(0.40) Per45 = "{:,.1f}".format(np.percentile(V, 45)) P45 = "{:.0%}".format(0.45) Per50 = "{:,.1f}".format(np.percentile(V, 50)) P50 = "{:.0%}".format(0.50) Per55 = "{:,.1f}".format(np.percentile(V, 55)) P55 = "{:.0%}".format(0.55) Per60 = "{:,.1f}".format(np.percentile(V, 60)) P60 = "{:.0%}".format(0.60) Per65 = "{:,.1f}".format(np.percentile(V, 65)) P65 = "{:.0%}".format(0.65) Per70 = "{:,.1f}".format(np.percentile(V, 70)) P70 = "{:.0%}".format(0.70) Per75 = "{:,.1f}".format(np.percentile(V, 75)) P75 = "{:.0%}".format(0.75) Per80 = "{:,.1f}".format(np.percentile(V, 80)) P80 = "{:.0%}".format(0.80) Per85 = "{:,.1f}".format(np.percentile(V, 85)) P85 = "{:.0%}".format(0.85) Per90 = "{:,.1f}".format(np.percentile(V, 90)) P90 = "{:.0%}".format(0.90) Per95 = "{:,.1f}".format(np.percentile(V, 95)) P95 = "{:.0%}".format(0.95) Minimum = "{:,.1f}".format(np.min(V)) Maximum = "{:,.1f}".format(np.max(V)) Mean = "{:,.1f}".format(np.mean(V)) Std_Dev = "{:,.1f}".format(np.std(V)) Variance = int(np.var(V)) Std_Error = "{:,.1f}".format(np.std(V)/np.sqrt(M)) Skewness = round(stats.skew(V),9) Kurtosis = round((stats.kurtosis(V)+3),9) Median = "{:,.1f}".format(np.median(V)) Mode = "{:,.1f}".format(stats.mode(V)[0][0]) Left_X = Per5 Left_P = P5 Right_X = Per95 Right_P = P95 Diff_X = "{:,.1f}".format((np.percentile(V, 95) - np.percentile(V, 5))) Diff_P = "{:.0%}".format(0.90) Confidence_Level = P95 Lower_Bound = "{:,.1f}".format((np.mean(V) - (np.std(V)/np.sqrt(M))*NORMSINV(0.975))) Upper_Bound = "{:,.1f}".format((np.mean(V) + (np.std(V)/np.sqrt(M))*NORMSINV(0.975))) g = {'Information': e, 'Result': f} st = pd.DataFrame(data=g) a = ['Minimum','Maximum','Mean','Std Dev','Variance','Std Error', 'Skewness','Kurtosis','Median','Mode',\'Left X','Left P','Right X','Right P','Diff X','Diff P','Confidence Level','Lower 95.0%','Upper 95.0%'] b = [Minimum, Maximum, Mean, Std_Dev, Variance, Std_Error, Skewness, Kurtosis, Median, Mode, Left_X, Left_P,\Right_X, Right_P, Diff_X, Diff_P, Confidence_Level, Lower_Bound, Upper_Bound] c = [P5,P10,P15,P20,P25,P30,P35,P40,P45,P50,P55,P60,P65,P70,P75,P80,P85,P90,P95] d = [Per5, Per10, Per15, Per20, Per25, Per30, Per35, Per40, Per45, Per50, Per55, Per60, Per65,\Per70, Per75, Per80, Per85, Per90, Per95] d = {'Statistics': a, 'Statistics Result': b, 'Percentile': c, 'Percentile Result': d} st1 = pd.DataFrame(data=d) from datetime import date today = date.today() now = datetime.now() import calendar curr_date = date.today() print("\033[1m Simulation Summary Information") print("\033[0m ================================================") print("\033[1m Performed By:","\033[0mIntrinsic Value Team #1") print("\033[1m Date:","\033[0m",calendar.day_name[curr_date.weekday()],",",today.strftime("%B %d, %Y"),",",now.strftime("%H:%M:%S AM")) st
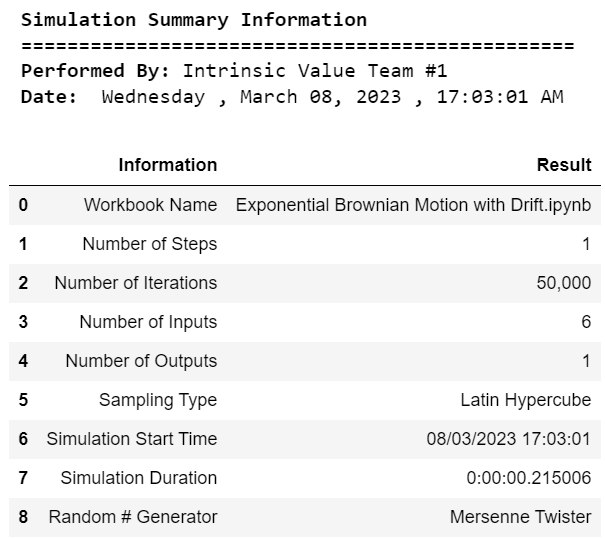
print("\033[1m Summary Statistics for Future Value of Stock") print("\033[0m ======================================================") print("\033[1m Performed By:","\033[0mIntrinsic Value Team #1") print("\033[1m Date:","\033[0m",calendar.day_name[curr_date.weekday()],",",today.strftime("%B %d, %Y"),",",now.strftime("%H:%M:%S AM")) st1
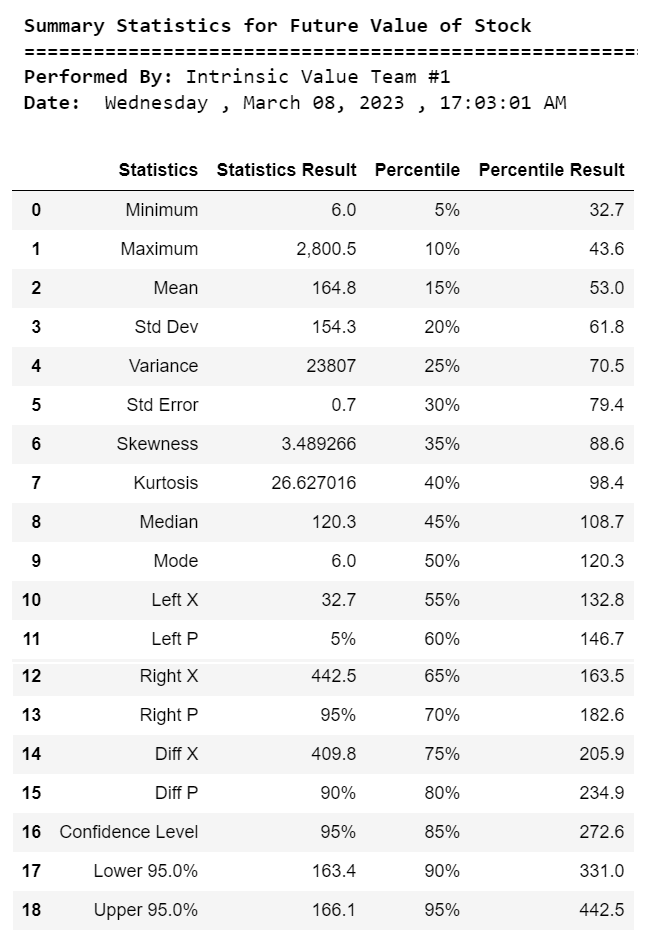
plt.figure(figsize = (4,4)) sns.set(font_scale = 1.2) sns.set_style('white') ax = sns.histplot(data=V,bins=50,color='red') ax.set_xlabel('Values',fontsize=14) ax.set_xlim( np.percentile(V, 0) , np.percentile(V, 99) ) print("\033[1m Probability Density Function for Future Value of Stock (Sim#1)") print("\033[0m ======================================================") print("\033[1m Performed By:","\033[0mIntrinsic Value Team #1") print("\033[1m Date:","\033[0m",calendar.day_name[curr_date.weekday()],",",today.strftime("%B %d, %Y"),",",now.strftime("%H:%M:%S AM")
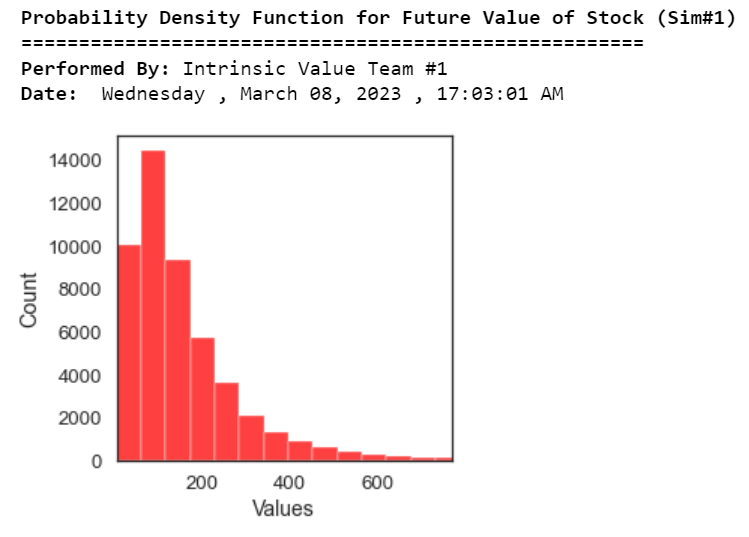
plt.figure(figsize = (4,4)) kwargs = {'cumulative': True} sns.set(font_scale = 1.2) sns.set_style('white') ax = sns.ecdfplot(V, color='red') ax.set_xlabel('Values',fontsize=14) ax.set_xlim( np.percentile(V, 0) , np.percentile(V, 99) ) print("\033[1m Cumulative Distribution Function for Future Value of Stock (Sim#1)") print("\033[0m ======================================================") print("\033[1m Performed By:","\033[0mIntrinsic Value Team #1") print("\033[1m Date:","\033[0m",calendar.day_name[curr_date.weekday()],",",today.strftime("%B %d, %Y"),",",now.strftime("%H:%M:%S AM"))
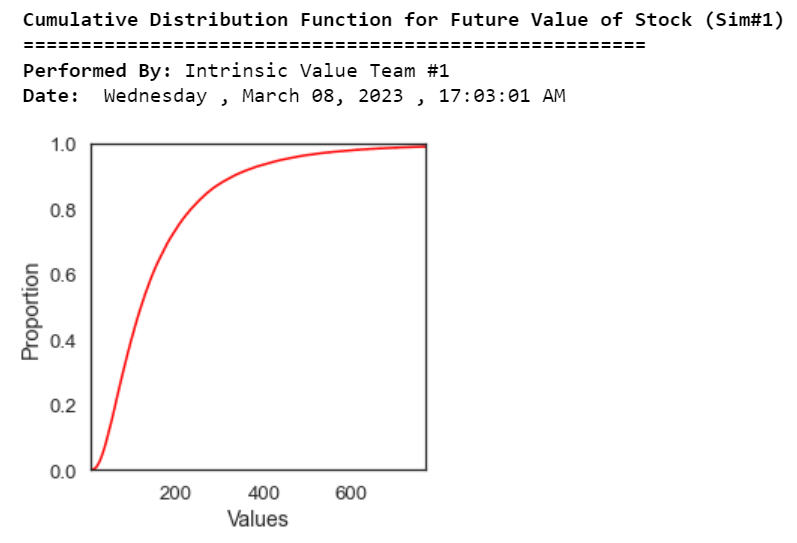
八、总结
蒙特卡罗模拟涉及使用随机数对股票价格在现实世界中可能遵循的许多不同路径(迭代)进行采样。对于每个路径,将计算未来值。未来价值的算术平均值是股票的预测未来价值。
样本均值的标准误差通常用作模型精度水平的指示。由于此标准误差随着路径数量的增加而下降非常缓慢,因此通常需要使用数万条(甚至更多)的样本路径才能达到可接受的精度水平。
参考:
Python 中具有漂移过程的指数布朗运动(随机游走) |中等 (medium.com)